An Algorithm Driven Sepsis Prediction Biomarker
Primary Purpose
Sepsis, Septic Shock, Severe Sepsis
Status
Completed
Phase
Not Applicable
Locations
United States
Study Type
Interventional
Intervention
Severe Sepsis Prediction
Severe Sepsis Detection
Sponsored by
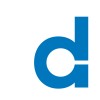
About this trial
This is an interventional screening trial for Sepsis focused on measuring InSight, Dascena
Eligibility Criteria
Inclusion Criteria:
- All adult patients admitted to the participating units will be eligible.
Exclusion Criteria:
- All patients younger than 18 years of age will be excluded.
Sites / Locations
- UCSF Moffit-Long Hospital
Arms of the Study
Arm 1
Arm 2
Arm Type
Experimental
Active Comparator
Arm Label
With InSight
Without InSight
Arm Description
Healthcare provider receives an alert from InSight for patients trending towards severe sepsis. Healthcare provider also receives information from the severe sepsis detector in the UCSF electronic health record.
Healthcare provider does not receive any alerts from InSight. Healthcare provider receives information from the severe sepsis detector in the UCSF electronic health record.
Outcomes
Primary Outcome Measures
Hospital length of stay
Secondary Outcome Measures
In-hospital mortality
Full Information
NCT ID
NCT03015454
First Posted
December 31, 2016
Last Updated
September 17, 2021
Sponsor
Dascena
Collaborators
University of California, San Francisco
1. Study Identification
Unique Protocol Identification Number
NCT03015454
Brief Title
An Algorithm Driven Sepsis Prediction Biomarker
Official Title
A Randomized Controlled Clinical Trial of an Algorithm Driven Sepsis Prediction Biomarker
Study Type
Interventional
2. Study Status
Record Verification Date
September 2021
Overall Recruitment Status
Completed
Study Start Date
December 2016 (undefined)
Primary Completion Date
February 2017 (Actual)
Study Completion Date
undefined (undefined)
3. Sponsor/Collaborators
Responsible Party, by Official Title
Sponsor
Name of the Sponsor
Dascena
Collaborators
University of California, San Francisco
4. Oversight
5. Study Description
Brief Summary
A sepsis early warning predictive algorithm, InSight, has been developed and validated on a large, diverse patient cohort. In this prospective study, the ability of InSight to predict severe sepsis patients is investigated. Specifically, InSight is compared with a well established severe sepsis detector in the UCSF electronic health record (EHR).
6. Conditions and Keywords
Primary Disease or Condition Being Studied in the Trial, or the Focus of the Study
Sepsis, Septic Shock, Severe Sepsis
Keywords
InSight, Dascena
7. Study Design
Primary Purpose
Screening
Study Phase
Not Applicable
Interventional Study Model
Factorial Assignment
Masking
None (Open Label)
Allocation
Randomized
Enrollment
142 (Actual)
8. Arms, Groups, and Interventions
Arm Title
With InSight
Arm Type
Experimental
Arm Description
Healthcare provider receives an alert from InSight for patients trending towards severe sepsis. Healthcare provider also receives information from the severe sepsis detector in the UCSF electronic health record.
Arm Title
Without InSight
Arm Type
Active Comparator
Arm Description
Healthcare provider does not receive any alerts from InSight. Healthcare provider receives information from the severe sepsis detector in the UCSF electronic health record.
Intervention Type
Other
Intervention Name(s)
Severe Sepsis Prediction
Intervention Description
Upon receiving an InSight alert, healthcare provider follows standard practices in assessing possible (severe) sepsis and intervening accordingly.
Intervention Type
Other
Intervention Name(s)
Severe Sepsis Detection
Intervention Description
Upon receiving information from the severe sepsis detector in the UCSF electronic health record, healthcare provider follows standard practices in assessing possible (severe) sepsis and intervening accordingly.
Primary Outcome Measure Information:
Title
Hospital length of stay
Time Frame
Through study completion, an average of 45 days
Secondary Outcome Measure Information:
Title
In-hospital mortality
Time Frame
Through study completion, an average of 45 days
Other Pre-specified Outcome Measures:
Title
ICU length of stay
Time Frame
Through study completion, an average of 45 days
10. Eligibility
Sex
All
Minimum Age & Unit of Time
18 Years
Accepts Healthy Volunteers
No
Eligibility Criteria
Inclusion Criteria:
All adult patients admitted to the participating units will be eligible.
Exclusion Criteria:
All patients younger than 18 years of age will be excluded.
Overall Study Officials:
First Name & Middle Initial & Last Name & Degree
Ritankar Das
Organizational Affiliation
Dascena
Official's Role
Principal Investigator
Facility Information:
Facility Name
UCSF Moffit-Long Hospital
City
San Francisco
State/Province
California
ZIP/Postal Code
94143
Country
United States
12. IPD Sharing Statement
Citations:
PubMed Identifier
27026611
Citation
Calvert JS, Price DA, Barton CW, Chettipally UK, Das R. Discharge recommendation based on a novel technique of homeostatic analysis. J Am Med Inform Assoc. 2017 Jan;24(1):24-29. doi: 10.1093/jamia/ocw014. Epub 2016 Mar 28.
Results Reference
background
PubMed Identifier
27489621
Citation
Calvert J, Desautels T, Chettipally U, Barton C, Hoffman J, Jay M, Mao Q, Mohamadlou H, Das R. High-performance detection and early prediction of septic shock for alcohol-use disorder patients. Ann Med Surg (Lond). 2016 May 10;8:50-5. doi: 10.1016/j.amsu.2016.04.023. eCollection 2016 Jun.
Results Reference
background
PubMed Identifier
27208704
Citation
Calvert JS, Price DA, Chettipally UK, Barton CW, Feldman MD, Hoffman JL, Jay M, Das R. A computational approach to early sepsis detection. Comput Biol Med. 2016 Jul 1;74:69-73. doi: 10.1016/j.compbiomed.2016.05.003. Epub 2016 May 12.
Results Reference
background
PubMed Identifier
27694098
Citation
Desautels T, Calvert J, Hoffman J, Jay M, Kerem Y, Shieh L, Shimabukuro D, Chettipally U, Feldman MD, Barton C, Wales DJ, Das R. Prediction of Sepsis in the Intensive Care Unit With Minimal Electronic Health Record Data: A Machine Learning Approach. JMIR Med Inform. 2016 Sep 30;4(3):e28. doi: 10.2196/medinform.5909.
Results Reference
background
PubMed Identifier
29435343
Citation
Shimabukuro DW, Barton CW, Feldman MD, Mataraso SJ, Das R. Effect of a machine learning-based severe sepsis prediction algorithm on patient survival and hospital length of stay: a randomised clinical trial. BMJ Open Respir Res. 2017 Nov 9;4(1):e000234. doi: 10.1136/bmjresp-2017-000234. eCollection 2017.
Results Reference
derived
Learn more about this trial
An Algorithm Driven Sepsis Prediction Biomarker
We'll reach out to this number within 24 hrs