Inpatient Mortality Prediction Algorithm Clinical Trial (IMPACT) (IMPACT)
Primary Purpose
Decompensation, Heart, Decompensation; Heart, Congestive, Death
Status
Withdrawn
Phase
Not Applicable
Locations
United States
Study Type
Interventional
Intervention
Patient mortality prediction
Sponsored by
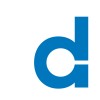
About this trial
This is an interventional diagnostic trial for Decompensation, Heart focused on measuring Dascena, patient mortality, machine learning, algorithm, diagnostic
Eligibility Criteria
Inclusion Criteria:
- All adult patients admitted to the participating units will be eligible.
Exclusion Criteria:
- All patients younger than 18 years of age will be excluded.
Sites / Locations
- UCSF Moffit-Long Hospital
Arms of the Study
Arm 1
Arm 2
Arm Type
Experimental
No Intervention
Arm Label
Prediction Algorithm
Control
Arm Description
Outcomes
Primary Outcome Measures
In-hospital mortality
Secondary Outcome Measures
Hospital length of stay
Full Information
NCT ID
NCT03212534
First Posted
July 6, 2017
Last Updated
September 20, 2021
Sponsor
Dascena
Collaborators
University of California, San Francisco
1. Study Identification
Unique Protocol Identification Number
NCT03212534
Brief Title
Inpatient Mortality Prediction Algorithm Clinical Trial (IMPACT)
Acronym
IMPACT
Official Title
A Randomized Clinical Trial of a Mortality Prediction Algorithm
Study Type
Interventional
2. Study Status
Record Verification Date
September 2021
Overall Recruitment Status
Withdrawn
Why Stopped
This trial did not start. No participants enrolled
Study Start Date
July 2017 (Anticipated)
Primary Completion Date
October 2017 (Anticipated)
Study Completion Date
October 2017 (Anticipated)
3. Sponsor/Collaborators
Responsible Party, by Official Title
Sponsor
Name of the Sponsor
Dascena
Collaborators
University of California, San Francisco
4. Oversight
Studies a U.S. FDA-regulated Drug Product
No
Studies a U.S. FDA-regulated Device Product
No
5. Study Description
Brief Summary
Through the mapping of retrospective patient data into a discrete multidimensional space, a novel algorithm for homeostatic analysis, was built to make outcome predictions. In this prospective study, the ability of the algorithm to predict patient mortality and influence clinical outcomes, will be investigated.
6. Conditions and Keywords
Primary Disease or Condition Being Studied in the Trial, or the Focus of the Study
Decompensation, Heart, Decompensation; Heart, Congestive, Death
Keywords
Dascena, patient mortality, machine learning, algorithm, diagnostic
7. Study Design
Primary Purpose
Diagnostic
Study Phase
Not Applicable
Interventional Study Model
Parallel Assignment
Masking
None (Open Label)
Allocation
Randomized
Enrollment
0 (Actual)
8. Arms, Groups, and Interventions
Arm Title
Prediction Algorithm
Arm Type
Experimental
Arm Title
Control
Arm Type
No Intervention
Intervention Type
Other
Intervention Name(s)
Patient mortality prediction
Intervention Description
Healthcare provider is notified of patient mortality prediction.
Primary Outcome Measure Information:
Title
In-hospital mortality
Time Frame
Through study completion, an average of 30 days
Secondary Outcome Measure Information:
Title
Hospital length of stay
Time Frame
Through study completion, an average of 30 days
Other Pre-specified Outcome Measures:
Title
Hospital readmission
Time Frame
Through study completion, an average of 30 days
Title
ICU length of stay
Time Frame
Through study completion, an average of 30 days
10. Eligibility
Sex
All
Minimum Age & Unit of Time
18 Years
Accepts Healthy Volunteers
No
Eligibility Criteria
Inclusion Criteria:
All adult patients admitted to the participating units will be eligible.
Exclusion Criteria:
All patients younger than 18 years of age will be excluded.
Overall Study Officials:
First Name & Middle Initial & Last Name & Degree
David Shimabukuro
Organizational Affiliation
University of California, San Francisco
Official's Role
Principal Investigator
Facility Information:
Facility Name
UCSF Moffit-Long Hospital
City
San Francisco
State/Province
California
ZIP/Postal Code
94143
Country
United States
12. IPD Sharing Statement
Citations:
PubMed Identifier
28638239
Citation
Desautels T, Calvert J, Hoffman J, Mao Q, Jay M, Fletcher G, Barton C, Chettipally U, Kerem Y, Das R. Using Transfer Learning for Improved Mortality Prediction in a Data-Scarce Hospital Setting. Biomed Inform Insights. 2017 Jun 12;9:1178222617712994. doi: 10.1177/1178222617712994. eCollection 2017.
Results Reference
background
PubMed Identifier
27253619
Citation
Calvert J, Mao Q, Rogers AJ, Barton C, Jay M, Desautels T, Mohamadlou H, Jan J, Das R. A computational approach to mortality prediction of alcohol use disorder inpatients. Comput Biol Med. 2016 Aug 1;75:74-9. doi: 10.1016/j.compbiomed.2016.05.015. Epub 2016 May 24.
Results Reference
background
PubMed Identifier
27026611
Citation
Calvert JS, Price DA, Barton CW, Chettipally UK, Das R. Discharge recommendation based on a novel technique of homeostatic analysis. J Am Med Inform Assoc. 2017 Jan;24(1):24-29. doi: 10.1093/jamia/ocw014. Epub 2016 Mar 28.
Results Reference
background
PubMed Identifier
27699003
Citation
Calvert J, Mao Q, Hoffman JL, Jay M, Desautels T, Mohamadlou H, Chettipally U, Das R. Using electronic health record collected clinical variables to predict medical intensive care unit mortality. Ann Med Surg (Lond). 2016 Sep 6;11:52-57. doi: 10.1016/j.amsu.2016.09.002. eCollection 2016 Nov.
Results Reference
background
Learn more about this trial
Inpatient Mortality Prediction Algorithm Clinical Trial (IMPACT)
We'll reach out to this number within 24 hrs