Unsupervised Machine Learning for Clustering of Septic Patients to Determine Optimal Treatment
Primary Purpose
Sepsis, Severe Sepsis, Septic Shock
Status
Not yet recruiting
Phase
Phase 2
Locations
Study Type
Interventional
Intervention
Treatment-specific InSight
InSight
Sponsored by
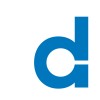
About this trial
This is an interventional diagnostic trial for Sepsis focused on measuring Dascena, machine learning, fluid administration, clustering algorithm, mortality, diagnostic
Eligibility Criteria
Inclusion Criteria:
- All adults above age 18 who are a member of one of the clinical subpopulations studied in this trial are eligible to participate in the study.
Exclusion Criteria:
- Under age 18
Sites / Locations
Arms of the Study
Arm 1
Arm 2
Arm Type
Experimental
Active Comparator
Arm Label
Fluid treatment-specific algorithm
Standard InSight
Arm Description
The experimental arm will involve patients monitored by the fluid treatment-customized version of InSight.
The control arm will involve patients monitored with the standard, non-treatment specific version of InSight.
Outcomes
Primary Outcome Measures
In-hospital SIRS-based mortality
Mortality attributed to patients meeting two or more SIRS criteria at some point during their stay
Secondary Outcome Measures
Full Information
1. Study Identification
Unique Protocol Identification Number
NCT03752489
Brief Title
Unsupervised Machine Learning for Clustering of Septic Patients to Determine Optimal Treatment
Official Title
Unsupervised Machine Learning for Clustering of Septic Patients to Determine Optimal Treatment
Study Type
Interventional
2. Study Status
Record Verification Date
September 2021
Overall Recruitment Status
Not yet recruiting
Study Start Date
April 1, 2022 (Anticipated)
Primary Completion Date
March 31, 2024 (Anticipated)
Study Completion Date
March 31, 2024 (Anticipated)
3. Sponsor/Collaborators
Responsible Party, by Official Title
Sponsor
Name of the Sponsor
Dascena
4. Oversight
Studies a U.S. FDA-regulated Drug Product
No
Studies a U.S. FDA-regulated Device Product
No
Data Monitoring Committee
Yes
5. Study Description
Brief Summary
The focus of this study will be to conduct a prospective, randomized controlled trial (RCT) at Cape Regional Medical Center (CRMC), Oroville Hospital (OH), and UCSF Medical Center (UCSF) in which a fluid treatment-specific algorithm will be applied to EHR data for the detection of severe sepsis. For patients determined to have a high risk of severe sepsis, the algorithm will generate automated voice, telephone notification to nursing staff at CRMC, OH, and UCSF. The algorithm's performance will be measured by analysis of the primary endpoint, reductions in in-hospital mortality.
6. Conditions and Keywords
Primary Disease or Condition Being Studied in the Trial, or the Focus of the Study
Sepsis, Severe Sepsis, Septic Shock
Keywords
Dascena, machine learning, fluid administration, clustering algorithm, mortality, diagnostic
7. Study Design
Primary Purpose
Diagnostic
Study Phase
Phase 2
Interventional Study Model
Parallel Assignment
Masking
ParticipantCare ProviderInvestigator
Allocation
Randomized
Enrollment
51645 (Anticipated)
8. Arms, Groups, and Interventions
Arm Title
Fluid treatment-specific algorithm
Arm Type
Experimental
Arm Description
The experimental arm will involve patients monitored by the fluid treatment-customized version of InSight.
Arm Title
Standard InSight
Arm Type
Active Comparator
Arm Description
The control arm will involve patients monitored with the standard, non-treatment specific version of InSight.
Intervention Type
Diagnostic Test
Intervention Name(s)
Treatment-specific InSight
Intervention Description
The InSight algorithm which draws information from a patient's electronic health record (EHR) to predict the onset of severe sepsis, and in this study will be customized to differentiate between clusters of patients who respond similarly to fluids treatment according to the nature of their disease progression.
Intervention Type
Diagnostic Test
Intervention Name(s)
InSight
Intervention Description
The non-customized InSight algorithm which draws information from a patient's electronic health record (EHR) to predict the onset of severe sepsis.
Primary Outcome Measure Information:
Title
In-hospital SIRS-based mortality
Description
Mortality attributed to patients meeting two or more SIRS criteria at some point during their stay
Time Frame
Through study completion, an average of 8 months
10. Eligibility
Sex
All
Minimum Age & Unit of Time
18 Years
Accepts Healthy Volunteers
Accepts Healthy Volunteers
Eligibility Criteria
Inclusion Criteria:
All adults above age 18 who are a member of one of the clinical subpopulations studied in this trial are eligible to participate in the study.
Exclusion Criteria:
Under age 18
Central Contact Person:
First Name & Middle Initial & Last Name or Official Title & Degree
Qingqing Mao, PhD
Phone
5108269508
Email
qmao@dascena.com
Overall Study Officials:
First Name & Middle Initial & Last Name & Degree
Qingqing Mao, PhD
Organizational Affiliation
Dascena, Inc.
Official's Role
Principal Investigator
12. IPD Sharing Statement
Citations:
PubMed Identifier
27699003
Citation
Calvert J, Mao Q, Hoffman JL, Jay M, Desautels T, Mohamadlou H, Chettipally U, Das R. Using electronic health record collected clinical variables to predict medical intensive care unit mortality. Ann Med Surg (Lond). 2016 Sep 6;11:52-57. doi: 10.1016/j.amsu.2016.09.002. eCollection 2016 Nov.
Results Reference
background
PubMed Identifier
29435343
Citation
Shimabukuro DW, Barton CW, Feldman MD, Mataraso SJ, Das R. Effect of a machine learning-based severe sepsis prediction algorithm on patient survival and hospital length of stay: a randomised clinical trial. BMJ Open Respir Res. 2017 Nov 9;4(1):e000234. doi: 10.1136/bmjresp-2017-000234. eCollection 2017.
Results Reference
background
PubMed Identifier
29374661
Citation
Mao Q, Jay M, Hoffman JL, Calvert J, Barton C, Shimabukuro D, Shieh L, Chettipally U, Fletcher G, Kerem Y, Zhou Y, Das R. Multicentre validation of a sepsis prediction algorithm using only vital sign data in the emergency department, general ward and ICU. BMJ Open. 2018 Jan 26;8(1):e017833. doi: 10.1136/bmjopen-2017-017833.
Results Reference
background
Learn more about this trial
Unsupervised Machine Learning for Clustering of Septic Patients to Determine Optimal Treatment
We'll reach out to this number within 24 hrs