RCT of Sepsis Machine Learning Algorithm
Primary Purpose
Sepsis, Severe Sepsis, Septic Shock
Status
Withdrawn
Phase
Phase 2
Locations
Study Type
Interventional
Intervention
InSight
Sponsored by
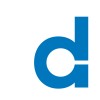
About this trial
This is an interventional diagnostic trial for Sepsis focused on measuring Dascena, patient mortality, machine learning, algorithm, diagnostic
Eligibility Criteria
Inclusion Criteria:
- During the study period, all patients over the age of 18 presenting to the emergency department or admitted to an inpatient unit at the participating facilities will automatically be enrolled in the trial, until the enrollment target for the study is met
Exclusion Criteria:
- Patients under the age of 18
Sites / Locations
Arms of the Study
Arm 1
Arm 2
Arm Type
Experimental
No Intervention
Arm Label
Experimental
Control
Arm Description
The experimental arm will involve patients monitored by InSight.
The control arm will have no intervention and will involve patients with the usual standard of care.
Outcomes
Primary Outcome Measures
In-hospital SIRS-based mortality
Rate of mortality attributed to patients meeting two or more SIRS criteria at some point during their stay
Secondary Outcome Measures
Full Information
NCT ID
NCT03882476
First Posted
March 18, 2019
Last Updated
September 17, 2021
Sponsor
Dascena
Collaborators
University of California, San Francisco
1. Study Identification
Unique Protocol Identification Number
NCT03882476
Brief Title
RCT of Sepsis Machine Learning Algorithm
Official Title
Randomized Controlled Trial of a Machine Learning Algorithm for Early Sepsis Detection
Study Type
Interventional
2. Study Status
Record Verification Date
September 2021
Overall Recruitment Status
Withdrawn
Why Stopped
Study not funded
Study Start Date
January 1, 2020 (Anticipated)
Primary Completion Date
February 28, 2021 (Anticipated)
Study Completion Date
February 28, 2021 (Anticipated)
3. Sponsor/Collaborators
Responsible Party, by Official Title
Sponsor
Name of the Sponsor
Dascena
Collaborators
University of California, San Francisco
4. Oversight
Studies a U.S. FDA-regulated Drug Product
No
Studies a U.S. FDA-regulated Device Product
No
5. Study Description
Brief Summary
The focus of this study will be to conduct a prospective, multi-center randomized controlled trial (RCT) at Cape Regional Medical Center (CRMC), Oroville Hospital (OH), and UCSF Medical Center (UCSF) in which a machine-learning algorithm will be applied to EHR data for the detection of sepsis. For patients determined to have a high risk of sepsis, the algorithm will generate automated voice, telephone notification to nursing staff at CRMC, OH, and UCSF. The algorithm's performance will be measured by analysis of the primary endpoint, in-hospital SIRS-based mortality.
Detailed Description
From January 2020 to February 2021, inclusive, investigators will perform a multi-center randomized controlled trial (RCT) at CRMC, OH, and UCSF. All aims of this study have been have been submitted for approval by the Pearl Institutional Review Board with a waiver of informed consent.
During the study period, all patients over the age of 18 presenting to the emergency department or admitted to an inpatient unit at the participating facilities will automatically be enrolled in the trial, until the target enrollment for the study is met. Enrollment will entail randomization to either the control or the experimental arms. Patients will be assigned to the experimental group or control group based on a random allocation sequence, generated by a computer program before the start of the trial, using simple randomization, with a 1:1 allocation ratio. This allocation sequence will be concealed to patients, healthcare providers and study investigators. However the trial will have an open-label design, as full blinding is not possible as some group assignments will become naturally revealed upon receipt of telephonic alerts.
There will be two arms in the study. The control arm will involve patients with the usual standard of care, and the experimental arm will involve patients monitored by InSight. If the applicable algorithm determines a patient to be at a high risk for sepsis, a telephonic alert will be sent to the charge nurse on duty in the patient's current location. Response to alerts will follow the protocol from our previous sepsis clinical trial. The procedure consists of a nurse conducting a patient bedside evaluation to rule out suspected infection. This includes assessment of patient vital signs, EHR notes, and recent laboratory results. If the nurse suspects sepsis, a physician subsequently assesses the patient and, if appropriate, places an order for administration of the standard sepsis treatment bundle.
In the administration of clinical trials, some open-label studies are cluster-randomized while others are randomized at an individual patient level. Cluster randomization is frequently used to minimize "contamination" between treatment and control groups, because exposure of providers to patients from both arms in an open-label study often invites unintentional behavioral biases. These biases may cause providers to adjust their interventions in the control group to mimic their actions in the experimental group, thereby masking the intervention's effect and skewing the study results towards the null. Although open-label, cluster-randomized trials are effective in minimizing contamination among groups, they have several significant disadvantages, including greater complexity in design and analysis as well as larger patient enrollment requirements to achieve the same statistical power. Because larger sample sizes often necessitate increases in cost, length, or complexity of a trial, current research has indicated that trialists should use individual randomization if possible due to the drawbacks of cluster allocation. Given these considerations, investigators concluded that individual randomization was the best strategy for the trial, as it affords a significant amount of increase in statistical power and allows each patient outcome to be assessed independently of every other patient. To minimize possible bias, investigators also decided to make the automated phone call text identical in both arms. The successful use of patient-level randomization in a previous sepsis clinical trial conducted by investigators gives confidence in this trial design.
After the discharge of the last enrolled patient, investigators will evaluate whether the primary endpoint of in-hospital SIRS-based mortality is met. Additional outcome measures of interest for each SIRS-based group will include: time to completion of each element of the Surviving Sepsis Campaign (SSC) bundle; ventilator-free days; ICU days; and 30-day hospital readmission rate. The 1-hour SSC bundle consists of obtaining blood cultures, measuring lactate level, administering broad-spectrum antibiotics, administering 30 mL/kg of crystalloid fluid for hypertension or lactate >4 mmol/L, and applying vasopressors if patient is hypotensive during or after fluid resuscitation.
Investigators plan to draw from EHR-based clinical data for primary endpoint analysis, as opposed to claims-based data, due to its ability to provide more objective measurements on patient outcomes.
At the conclusion of the study, significant findings will be published as scientific papers.
6. Conditions and Keywords
Primary Disease or Condition Being Studied in the Trial, or the Focus of the Study
Sepsis, Severe Sepsis, Septic Shock
Keywords
Dascena, patient mortality, machine learning, algorithm, diagnostic
7. Study Design
Primary Purpose
Diagnostic
Study Phase
Phase 2
Interventional Study Model
Parallel Assignment
Masking
ParticipantCare ProviderInvestigator
Allocation
Randomized
Enrollment
0 (Actual)
8. Arms, Groups, and Interventions
Arm Title
Experimental
Arm Type
Experimental
Arm Description
The experimental arm will involve patients monitored by InSight.
Arm Title
Control
Arm Type
No Intervention
Arm Description
The control arm will have no intervention and will involve patients with the usual standard of care.
Intervention Type
Diagnostic Test
Intervention Name(s)
InSight
Intervention Description
Clinical decision support (CDS) system for sepsis detection
Primary Outcome Measure Information:
Title
In-hospital SIRS-based mortality
Description
Rate of mortality attributed to patients meeting two or more SIRS criteria at some point during their stay
Time Frame
Through study completion, an average of eight months
10. Eligibility
Sex
All
Minimum Age & Unit of Time
18 Years
Accepts Healthy Volunteers
Accepts Healthy Volunteers
Eligibility Criteria
Inclusion Criteria:
During the study period, all patients over the age of 18 presenting to the emergency department or admitted to an inpatient unit at the participating facilities will automatically be enrolled in the trial, until the enrollment target for the study is met
Exclusion Criteria:
Patients under the age of 18
Overall Study Officials:
First Name & Middle Initial & Last Name & Degree
Ritankar Das, MSc
Organizational Affiliation
Dascena
Official's Role
Principal Investigator
12. IPD Sharing Statement
Citations:
PubMed Identifier
28638239
Citation
Desautels T, Calvert J, Hoffman J, Mao Q, Jay M, Fletcher G, Barton C, Chettipally U, Kerem Y, Das R. Using Transfer Learning for Improved Mortality Prediction in a Data-Scarce Hospital Setting. Biomed Inform Insights. 2017 Jun 12;9:1178222617712994. doi: 10.1177/1178222617712994. eCollection 2017.
Results Reference
background
PubMed Identifier
27253619
Citation
Calvert J, Mao Q, Rogers AJ, Barton C, Jay M, Desautels T, Mohamadlou H, Jan J, Das R. A computational approach to mortality prediction of alcohol use disorder inpatients. Comput Biol Med. 2016 Aug 1;75:74-9. doi: 10.1016/j.compbiomed.2016.05.015. Epub 2016 May 24.
Results Reference
background
PubMed Identifier
27026611
Citation
Calvert JS, Price DA, Barton CW, Chettipally UK, Das R. Discharge recommendation based on a novel technique of homeostatic analysis. J Am Med Inform Assoc. 2017 Jan;24(1):24-29. doi: 10.1093/jamia/ocw014. Epub 2016 Mar 28.
Results Reference
background
PubMed Identifier
27699003
Citation
Calvert J, Mao Q, Hoffman JL, Jay M, Desautels T, Mohamadlou H, Chettipally U, Das R. Using electronic health record collected clinical variables to predict medical intensive care unit mortality. Ann Med Surg (Lond). 2016 Sep 6;11:52-57. doi: 10.1016/j.amsu.2016.09.002. eCollection 2016 Nov.
Results Reference
background
PubMed Identifier
29435343
Citation
Shimabukuro DW, Barton CW, Feldman MD, Mataraso SJ, Das R. Effect of a machine learning-based severe sepsis prediction algorithm on patient survival and hospital length of stay: a randomised clinical trial. BMJ Open Respir Res. 2017 Nov 9;4(1):e000234. doi: 10.1136/bmjresp-2017-000234. eCollection 2017.
Results Reference
background
Learn more about this trial
RCT of Sepsis Machine Learning Algorithm
We'll reach out to this number within 24 hrs