Build-up Computed Assisted History Taking, Physical Examination and Diagnosis System of Emergency Patient Through Machine Learning (II) (MLD)
Primary Purpose
Internal Disease
Status
Recruiting
Phase
Not Applicable
Locations
Taiwan
Study Type
Interventional
Intervention
Artificial intelligence
Sponsored by
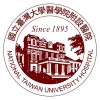
About this trial
This is an interventional treatment trial for Internal Disease focused on measuring Artificial intelligence
Eligibility Criteria
Inclusion Criteria:
- Over twenty years old
- Non-traumatic patient
Exclusion Criteria:
- Excluding the patients for administration reasons (issuing a medical certificate)
- Excluding the patients for non-emergency reasons like simply acupuncture, virus screening and prescription for medication.
- Excluding Patients who allocated to critical care station
Sites / Locations
- National Taiwan University HospitalRecruiting
Arms of the Study
Arm 1
Arm 2
Arm Type
No Intervention
Experimental
Arm Label
Control
Experimental
Arm Description
Outcomes
Primary Outcome Measures
Senior doctor appraisal
Senior doctor appraisal which measured by an established questionnaire. Senior doctor will fill an expert-verified clinical note quality evaluation questionnaire after junior doctor finished patient interview and clinical note recording. The questionnaire is designed to use 5 points likert scale and higher scores mean a better outcome.
Secondary Outcome Measures
Accuracy of diagnosis prediction
The percentage of predicted diagnosis match the final diagnosis.
Rationality of diagnosis prediction
Senior doctors will assess rationality of predicted diagnosis.
Full Information
NCT ID
NCT05596929
First Posted
October 10, 2022
Last Updated
February 20, 2023
Sponsor
National Taiwan University Hospital
1. Study Identification
Unique Protocol Identification Number
NCT05596929
Brief Title
Build-up Computed Assisted History Taking, Physical Examination and Diagnosis System of Emergency Patient Through Machine Learning (II)
Acronym
MLD
Official Title
Build-up Computed Assisted History Taking, Physical Examination and Diagnosis System of Emergency Patient Through Machine Learning (II)
Study Type
Interventional
2. Study Status
Record Verification Date
December 2022
Overall Recruitment Status
Recruiting
Study Start Date
December 12, 2022 (Actual)
Primary Completion Date
March 30, 2023 (Anticipated)
Study Completion Date
March 30, 2023 (Anticipated)
3. Sponsor/Collaborators
Responsible Party, by Official Title
Sponsor
Name of the Sponsor
National Taiwan University Hospital
4. Oversight
Studies a U.S. FDA-regulated Drug Product
No
Studies a U.S. FDA-regulated Device Product
No
Data Monitoring Committee
No
5. Study Description
Brief Summary
In emergency department(ED), physicians need to complete patient evaluation and management in a short time, which required different history taking, and physical examination skill in healthcare system.
Natural language processing(NLP) became easily accessible after the development of machine learning(ML). Besides, electronic medical record(EMR) had been widely applied in healthcare systems. There are more and more tools try to capture certain information from the EMR help clinical workers handle increasing patient data and improving patient care.
However, to err is human. Physicians might omit some important signs or symptoms, or forget to write it down in the record especially in a busy emergency room. It will lead to an unfavorable outcome when there were medical legal issue or national health insurance review. The condition could be limited by a EMR supporting system. The quality of care will also improve.
The investigators are planning to analyze EMR of emergency room by NLP and machine learning. To establish the linkage between triage data, chief complaint, past history, present illness and physical examination. The investigators will try to predict the tentative diagnosis and patient disposition after the relationship being found. Thereafter, the investigators could try to predict the key element of history taking and physical examination of the patient and inform the physician when the miss happened. The investigators hope the system may improve the quality of medical recording and patient care.
6. Conditions and Keywords
Primary Disease or Condition Being Studied in the Trial, or the Focus of the Study
Internal Disease
Keywords
Artificial intelligence
7. Study Design
Primary Purpose
Treatment
Study Phase
Not Applicable
Interventional Study Model
Parallel Assignment
Masking
ParticipantCare ProviderInvestigator
Allocation
Randomized
Enrollment
3000 (Anticipated)
8. Arms, Groups, and Interventions
Arm Title
Control
Arm Type
No Intervention
Arm Title
Experimental
Arm Type
Experimental
Intervention Type
Diagnostic Test
Intervention Name(s)
Artificial intelligence
Intervention Description
After the patients under triage classification to which randomly allocates in two groups. The group with AI intervention and the other without AI intervention.
Primary Outcome Measure Information:
Title
Senior doctor appraisal
Description
Senior doctor appraisal which measured by an established questionnaire. Senior doctor will fill an expert-verified clinical note quality evaluation questionnaire after junior doctor finished patient interview and clinical note recording. The questionnaire is designed to use 5 points likert scale and higher scores mean a better outcome.
Time Frame
24 hours
Secondary Outcome Measure Information:
Title
Accuracy of diagnosis prediction
Description
The percentage of predicted diagnosis match the final diagnosis.
Time Frame
patient discharge from ED, up to 1 week
Title
Rationality of diagnosis prediction
Description
Senior doctors will assess rationality of predicted diagnosis.
Time Frame
24 hours
10. Eligibility
Sex
All
Minimum Age & Unit of Time
20 Years
Accepts Healthy Volunteers
No
Eligibility Criteria
Inclusion Criteria:
Over twenty years old
Non-traumatic patient
Exclusion Criteria:
Excluding the patients for administration reasons (issuing a medical certificate)
Excluding the patients for non-emergency reasons like simply acupuncture, virus screening and prescription for medication.
Excluding Patients who allocated to critical care station
Central Contact Person:
First Name & Middle Initial & Last Name or Official Title & Degree
Hui-Chih Wang, Dr.
Phone
+88623123456
Email
ticoer@ntuh.gov.tw
First Name & Middle Initial & Last Name or Official Title & Degree
Hsin-Hsi Chen, Dr.
Phone
+886233664888
Ext
311
Email
hhchen@ntu.edu.tw
Overall Study Officials:
First Name & Middle Initial & Last Name & Degree
Huang, Dr.
Organizational Affiliation
National Taiwan University Hospital
Official's Role
Study Chair
Facility Information:
Facility Name
National Taiwan University Hospital
City
Taipei
ZIP/Postal Code
100
Country
Taiwan
Individual Site Status
Recruiting
Facility Contact:
First Name & Middle Initial & Last Name & Degree
Wang, Dr.
Phone
886-2-23123456
Ext
265659
Email
ticoer@ntuh.gov.tw
12. IPD Sharing Statement
Plan to Share IPD
No
Learn more about this trial
Build-up Computed Assisted History Taking, Physical Examination and Diagnosis System of Emergency Patient Through Machine Learning (II)
We'll reach out to this number within 24 hrs