Glaucoma Screening With Artificial Intelligence
Primary Purpose
Glaucoma
Status
Recruiting
Phase
Not Applicable
Locations
Hong Kong
Study Type
Interventional
Intervention
ROTA assessment by AI
Optic disc assessment by AI
Sponsored by
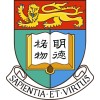
About this trial
This is an interventional screening trial for Glaucoma focused on measuring Ophthalmology, Glaucoma, Artificial Intelligence, Retinal Nerve Fiber Layer Optical Texture Analysis, Optic Disc Photography Assessment
Eligibility Criteria
Inclusion Criteria: Individuals aged 50 years or above Exclusion Criteria: Physically incapacitated Not able to cooperate for clinical examination or optical coherence tomography (OCT) investigation will be excluded
Sites / Locations
- Southern District Wah Kwai Community CentreRecruiting
- Kwun Tong District Health CentreRecruiting
Arms of the Study
Arm 1
Arm 2
Arm Type
Experimental
Active Comparator
Arm Label
Retinal nerve fiber layer optical texture analysis (ROTA)
Optic disc photography
Arm Description
The RNFL is imaged with OCT for ROTA.
The optic disc is imaged with color fundus camera.
Outcomes
Primary Outcome Measures
Diagnostic performance for detection of glaucoma
The area under the receiver operating characteristic curve (AUC) for detection of glaucoma
Secondary Outcome Measures
Incremental cost-effectiveness ratios (ICERs) for population screening of glaucoma
ICER for glaucoma screening measured by incremental cost per true positive case detected, incremental cost per incremental QALY
The prevalence of glaucoma
Proportion of patients with glaucoma
Full Information
NCT ID
NCT06012058
First Posted
August 21, 2023
Last Updated
September 19, 2023
Sponsor
The University of Hong Kong
Collaborators
Orbis
1. Study Identification
Unique Protocol Identification Number
NCT06012058
Brief Title
Glaucoma Screening With Artificial Intelligence
Official Title
Glaucoma Screening With Artificial Intelligence - A Randomized Clinical Trial Comparing Retinal Nerve Fiber Layer Optical Texture Analysis and Optic Disc Photography Assessment
Study Type
Interventional
2. Study Status
Record Verification Date
September 2023
Overall Recruitment Status
Recruiting
Study Start Date
August 26, 2023 (Actual)
Primary Completion Date
August 25, 2024 (Anticipated)
Study Completion Date
February 25, 2025 (Anticipated)
3. Sponsor/Collaborators
Responsible Party, by Official Title
Principal Investigator
Name of the Sponsor
The University of Hong Kong
Collaborators
Orbis
4. Oversight
Studies a U.S. FDA-regulated Drug Product
No
Studies a U.S. FDA-regulated Device Product
No
5. Study Description
Brief Summary
This randomized clinical trial aims to compare the diagnostic performance of two AI-enabled screening strategies - ROTA (RNFL optical texture analysis) assessment versus optic disc photography - in detecting glaucoma within a population-based sample. Secondary objectives are to (1) compare the diagnostic performance of ROTA AI assessment versus OCT RNFL thickness assessment by AI, and ROTA AI assessment versus OCT RNFL thickness assessment by trained graders, (2) investigate the cost-effectiveness of AI ROTA assessment for glaucoma screening, and (3) estimate the prevalence of glaucoma in Hong Kong.
Detailed Description
Glaucoma is the leading cause of irreversible blindness affecting 76 million patients worldwide in 2020. Characterized by progressive degeneration of the optic nerve, early detection of disease deterioration with timely intervention is critical to prevent progressive loss in vision. In the 5th World Glaucoma Association Consensus Meeting, a diverse and representative group of glaucoma clinicians and scientists deliberated on the value and methods of glaucoma screening. Whereas it has been recognized that early detection of glaucoma for treatment is beneficial to preserve the quality of vision and quality of life as glaucoma treatments are often effective, easy to use and well tolerated, the optimal screening strategy for glaucoma has not yet been determined.
ROTA (Retinal Nerve Fiber Layer Optical Texture Analysis) is a patented algorithm designed to detect axonal fiber bundle loss in glaucoma. Unlike conventional Optical Coherence Tomography (OCT) analysis, ROTA uses non-linear transformation to reveal the optical textures and trajectories of axonal fiber bundles, allowing for intuitive and reliable recognition of RNFL abnormalities without the need for normative databases. It can be applied across different OCT models and is particularly effective at detecting focal RNFL defects in early glaucoma and varying degrees of RNFL damage in end-stage glaucoma. The proposed study will address whether the application AI on ROTA is feasible and cost-effective in the setting of glaucoma screening, and whether ROTA would outperform optic disc photography and OCT RNFL thickness assessment.
6. Conditions and Keywords
Primary Disease or Condition Being Studied in the Trial, or the Focus of the Study
Glaucoma
Keywords
Ophthalmology, Glaucoma, Artificial Intelligence, Retinal Nerve Fiber Layer Optical Texture Analysis, Optic Disc Photography Assessment
7. Study Design
Primary Purpose
Screening
Study Phase
Not Applicable
Interventional Study Model
Parallel Assignment
Model Description
This is a randomized clinical trial with the primary objective to compare the diagnostic performance of two screening strategies - Retinal nerve fiber layer Optical Texture Analysis (ROTA) assessment by Artificial Intelligence (AI) versus (vs.) optic disc photography assessment by AI or trained graders - for detection of glaucoma in a population-based sample.
Masking
None (Open Label)
Allocation
Randomized
Enrollment
3175 (Anticipated)
8. Arms, Groups, and Interventions
Arm Title
Retinal nerve fiber layer optical texture analysis (ROTA)
Arm Type
Experimental
Arm Description
The RNFL is imaged with OCT for ROTA.
Arm Title
Optic disc photography
Arm Type
Active Comparator
Arm Description
The optic disc is imaged with color fundus camera.
Intervention Type
Diagnostic Test
Intervention Name(s)
ROTA assessment by AI
Intervention Description
The RNFL is imaged with OCT for ROTA and the data are analyzed with a deep learning model.
Intervention Type
Diagnostic Test
Intervention Name(s)
Optic disc assessment by AI
Intervention Description
The optic disc is imaged with color fundus camera and the data are analyzed with a deep learning model.
Primary Outcome Measure Information:
Title
Diagnostic performance for detection of glaucoma
Description
The area under the receiver operating characteristic curve (AUC) for detection of glaucoma
Time Frame
up to ~1 year
Secondary Outcome Measure Information:
Title
Incremental cost-effectiveness ratios (ICERs) for population screening of glaucoma
Description
ICER for glaucoma screening measured by incremental cost per true positive case detected, incremental cost per incremental QALY
Time Frame
up to ~1 year
Title
The prevalence of glaucoma
Description
Proportion of patients with glaucoma
Time Frame
up to ~1 year
Other Pre-specified Outcome Measures:
Title
Diagnostic performance for detection of macular diseases
Description
The area under the receiver operating characteristic curve (AUC) for detection of macular diseases
Time Frame
up to ~1 year
Title
Incremental cost-effectiveness ratios (ICERs) for population screening of glaucoma and macular diseases
Description
ICER for glaucoma and macular diseases screening measured by incremental cost per true positive case detected, incremental cost per incremental QALY
Time Frame
up to ~1 year
Title
The prevalence of macular diseases
Description
Proportion of patients with macular diseases
Time Frame
up to ~1 year
10. Eligibility
Sex
All
Minimum Age & Unit of Time
50 Years
Accepts Healthy Volunteers
Accepts Healthy Volunteers
Eligibility Criteria
Inclusion Criteria:
Individuals aged 50 years or above
Exclusion Criteria:
Physically incapacitated
Not able to cooperate for clinical examination or optical coherence tomography (OCT) investigation will be excluded
Central Contact Person:
First Name & Middle Initial & Last Name or Official Title & Degree
Anita Yau
Phone
39102673
Email
anitayky@hku.hk
Overall Study Officials:
First Name & Middle Initial & Last Name & Degree
Christopher Leung
Organizational Affiliation
The University of Hong Kong
Official's Role
Principal Investigator
Facility Information:
Facility Name
Southern District Wah Kwai Community Centre
City
Aberdeen
Country
Hong Kong
Individual Site Status
Recruiting
Facility Contact:
First Name & Middle Initial & Last Name & Degree
Jordy Lau
Phone
28315367
Email
jordylau@hku.hk
Facility Name
Kwun Tong District Health Centre
City
Kwun Tong
Country
Hong Kong
Individual Site Status
Recruiting
Facility Contact:
First Name & Middle Initial & Last Name & Degree
Jordy Lau
Phone
28315367
Email
jordylau@hku.hk
12. IPD Sharing Statement
Plan to Share IPD
No
Citations:
PubMed Identifier
29032195
Citation
Flaxman SR, Bourne RRA, Resnikoff S, Ackland P, Braithwaite T, Cicinelli MV, Das A, Jonas JB, Keeffe J, Kempen JH, Leasher J, Limburg H, Naidoo K, Pesudovs K, Silvester A, Stevens GA, Tahhan N, Wong TY, Taylor HR; Vision Loss Expert Group of the Global Burden of Disease Study. Global causes of blindness and distance vision impairment 1990-2020: a systematic review and meta-analysis. Lancet Glob Health. 2017 Dec;5(12):e1221-e1234. doi: 10.1016/S2214-109X(17)30393-5. Epub 2017 Oct 11.
Results Reference
background
PubMed Identifier
24974815
Citation
Tham YC, Li X, Wong TY, Quigley HA, Aung T, Cheng CY. Global prevalence of glaucoma and projections of glaucoma burden through 2040: a systematic review and meta-analysis. Ophthalmology. 2014 Nov;121(11):2081-90. doi: 10.1016/j.ophtha.2014.05.013. Epub 2014 Jun 26.
Results Reference
background
PubMed Identifier
27654570
Citation
Weinreb RN, Leung CK, Crowston JG, Medeiros FA, Friedman DS, Wiggs JL, Martin KR. Primary open-angle glaucoma. Nat Rev Dis Primers. 2016 Sep 22;2:16067. doi: 10.1038/nrdp.2016.67.
Results Reference
background
PubMed Identifier
19429591
Citation
Kim JS, Ishikawa H, Sung KR, Xu J, Wollstein G, Bilonick RA, Gabriele ML, Kagemann L, Duker JS, Fujimoto JG, Schuman JS. Retinal nerve fibre layer thickness measurement reproducibility improved with spectral domain optical coherence tomography. Br J Ophthalmol. 2009 Aug;93(8):1057-63. doi: 10.1136/bjo.2009.157875. Epub 2009 May 7.
Results Reference
background
PubMed Identifier
19464061
Citation
Leung CK, Cheung CY, Weinreb RN, Qiu Q, Liu S, Li H, Xu G, Fan N, Huang L, Pang CP, Lam DS. Retinal nerve fiber layer imaging with spectral-domain optical coherence tomography: a variability and diagnostic performance study. Ophthalmology. 2009 Jul;116(7):1257-63, 1263.e1-2. doi: 10.1016/j.ophtha.2009.04.013. Epub 2009 May 22.
Results Reference
background
PubMed Identifier
22871835
Citation
Pierro L, Gagliardi M, Iuliano L, Ambrosi A, Bandello F. Retinal nerve fiber layer thickness reproducibility using seven different OCT instruments. Invest Ophthalmol Vis Sci. 2012 Aug 31;53(9):5912-20. doi: 10.1167/iovs.11-8644.
Results Reference
background
PubMed Identifier
20663563
Citation
Leung CK, Lam S, Weinreb RN, Liu S, Ye C, Liu L, He J, Lai GW, Li T, Lam DS. Retinal nerve fiber layer imaging with spectral-domain optical coherence tomography: analysis of the retinal nerve fiber layer map for glaucoma detection. Ophthalmology. 2010 Sep;117(9):1684-91. doi: 10.1016/j.ophtha.2010.01.026. Epub 2010 Jul 21.
Results Reference
background
PubMed Identifier
20678802
Citation
Leung CK, Choi N, Weinreb RN, Liu S, Ye C, Liu L, Lai GW, Lau J, Lam DS. Retinal nerve fiber layer imaging with spectral-domain optical coherence tomography: pattern of RNFL defects in glaucoma. Ophthalmology. 2010 Dec;117(12):2337-44. doi: 10.1016/j.ophtha.2010.04.002. Epub 2010 Aug 3.
Results Reference
background
PubMed Identifier
22677426
Citation
Leung CK, Yu M, Weinreb RN, Lai G, Xu G, Lam DS. Retinal nerve fiber layer imaging with spectral-domain optical coherence tomography: patterns of retinal nerve fiber layer progression. Ophthalmology. 2012 Sep;119(9):1858-66. doi: 10.1016/j.ophtha.2012.03.044. Epub 2012 Jun 5.
Results Reference
background
PubMed Identifier
24053994
Citation
Xu G, Weinreb RN, Leung CKS. Retinal nerve fiber layer progression in glaucoma: a comparison between retinal nerve fiber layer thickness and retardance. Ophthalmology. 2013 Dec;120(12):2493-2500. doi: 10.1016/j.ophtha.2013.07.027. Epub 2013 Sep 17.
Results Reference
background
PubMed Identifier
25108319
Citation
Xu G, Weinreb RN, Leung CK. Optic nerve head deformation in glaucoma: the temporal relationship between optic nerve head surface depression and retinal nerve fiber layer thinning. Ophthalmology. 2014 Dec;121(12):2362-70. doi: 10.1016/j.ophtha.2014.06.035. Epub 2014 Aug 6.
Results Reference
background
PubMed Identifier
26891880
Citation
Oddone F, Lucenteforte E, Michelessi M, Rizzo S, Donati S, Parravano M, Virgili G. Macular versus Retinal Nerve Fiber Layer Parameters for Diagnosing Manifest Glaucoma: A Systematic Review of Diagnostic Accuracy Studies. Ophthalmology. 2016 May;123(5):939-49. doi: 10.1016/j.ophtha.2015.12.041. Epub 2016 Feb 15.
Results Reference
background
PubMed Identifier
27442185
Citation
Biswas S, Lin C, Leung CK. Evaluation of a Myopic Normative Database for Analysis of Retinal Nerve Fiber Layer Thickness. JAMA Ophthalmol. 2016 Sep 1;134(9):1032-9. doi: 10.1001/jamaophthalmol.2016.2343. Erratum In: JAMA Ophthalmol. 2016 Nov 1;134(11):1336.
Results Reference
background
PubMed Identifier
17122099
Citation
Leung CK, Mohamed S, Leung KS, Cheung CY, Chan SL, Cheng DK, Lee AK, Leung GY, Rao SK, Lam DS. Retinal nerve fiber layer measurements in myopia: An optical coherence tomography study. Invest Ophthalmol Vis Sci. 2006 Dec;47(12):5171-6. doi: 10.1167/iovs.06-0545.
Results Reference
background
PubMed Identifier
34992272
Citation
Leung CKS, Lam AKN, Weinreb RN, Garway-Heath DF, Yu M, Guo PY, Chiu VSM, Wan KHN, Wong M, Wu KZ, Cheung CYL, Lin C, Chan CKM, Chan NCY, Kam KW, Lai GWK. Diagnostic assessment of glaucoma and non-glaucomatous optic neuropathies via optical texture analysis of the retinal nerve fibre layer. Nat Biomed Eng. 2022 May;6(5):593-604. doi: 10.1038/s41551-021-00813-x. Epub 2022 Jan 6.
Results Reference
background
PubMed Identifier
31147377
Citation
Zheng F, Yu M, Leung CK. Diagnostic criteria for detection of retinal nerve fibre layer thickness and neuroretinal rim width abnormalities in glaucoma. Br J Ophthalmol. 2020 Feb;104(2):270-275. doi: 10.1136/bjophthalmol-2018-313581. Epub 2019 May 30.
Results Reference
background
PubMed Identifier
34325853
Citation
Lin D, Xiong J, Liu C, Zhao L, Li Z, Yu S, Wu X, Ge Z, Hu X, Wang B, Fu M, Zhao X, Wang X, Zhu Y, Chen C, Li T, Li Y, Wei W, Zhao M, Li J, Xu F, Ding L, Tan G, Xiang Y, Hu Y, Zhang P, Han Y, Li JO, Wei L, Zhu P, Liu Y, Chen W, Ting DSW, Wong TY, Chen Y, Lin H. Application of Comprehensive Artificial intelligence Retinal Expert (CARE) system: a national real-world evidence study. Lancet Digit Health. 2021 Aug;3(8):e486-e495. doi: 10.1016/S2589-7500(21)00086-8.
Results Reference
background
PubMed Identifier
29506863
Citation
Li Z, He Y, Keel S, Meng W, Chang RT, He M. Efficacy of a Deep Learning System for Detecting Glaucomatous Optic Neuropathy Based on Color Fundus Photographs. Ophthalmology. 2018 Aug;125(8):1199-1206. doi: 10.1016/j.ophtha.2018.01.023. Epub 2018 Mar 2.
Results Reference
background
PubMed Identifier
31513266
Citation
Liu H, Li L, Wormstone IM, Qiao C, Zhang C, Liu P, Li S, Wang H, Mou D, Pang R, Yang D, Zangwill LM, Moghimi S, Hou H, Bowd C, Jiang L, Chen Y, Hu M, Xu Y, Kang H, Ji X, Chang R, Tham C, Cheung C, Ting DSW, Wong TY, Wang Z, Weinreb RN, Xu M, Wang N. Development and Validation of a Deep Learning System to Detect Glaucomatous Optic Neuropathy Using Fundus Photographs. JAMA Ophthalmol. 2019 Dec 1;137(12):1353-1360. doi: 10.1001/jamaophthalmol.2019.3501. Erratum In: JAMA Ophthalmol. 2019 Dec 1;137(12):1468.
Results Reference
background
PubMed Identifier
16799014
Citation
He M, Foster PJ, Ge J, Huang W, Zheng Y, Friedman DS, Lee PS, Khaw PT. Prevalence and clinical characteristics of glaucoma in adult Chinese: a population-based study in Liwan District, Guangzhou. Invest Ophthalmol Vis Sci. 2006 Jul;47(7):2782-8. doi: 10.1167/iovs.06-0051.
Results Reference
background
PubMed Identifier
29433852
Citation
Hou HW, Lin C, Leung CK. Integrating Macular Ganglion Cell Inner Plexiform Layer and Parapapillary Retinal Nerve Fiber Layer Measurements to Detect Glaucoma Progression. Ophthalmology. 2018 Jun;125(6):822-831. doi: 10.1016/j.ophtha.2017.12.027. Epub 2018 Feb 9.
Results Reference
background
PubMed Identifier
27001534
Citation
Yu M, Lin C, Weinreb RN, Lai G, Chiu V, Leung CK. Risk of Visual Field Progression in Glaucoma Patients with Progressive Retinal Nerve Fiber Layer Thinning: A 5-Year Prospective Study. Ophthalmology. 2016 Jun;123(6):1201-10. doi: 10.1016/j.ophtha.2016.02.017. Epub 2016 Mar 19.
Results Reference
background
PubMed Identifier
32423768
Citation
Wu K, Lin C, Lam AK, Chan L, Leung CK. Wide-field Trend-based Progression Analysis of Combined Retinal Nerve Fiber Layer and Ganglion Cell Inner Plexiform Layer Thickness: A New Paradigm to Improve Glaucoma Progression Detection. Ophthalmology. 2020 Oct;127(10):1322-1330. doi: 10.1016/j.ophtha.2020.03.019. Epub 2020 Mar 29.
Results Reference
background
Links:
URL
https://epdf.tips/glaucoma-screening.html
Description
Glaucoma Screening, Consensus Series - 5. Hague, Netherlands: Kugler Publications, 2008.
URL
https://kugler.pub/catalogue/ophthalmology/wga-consensus-series/wga-consensus-series-10-diagnosis-of-primary-open-angle-glaucoma/
Description
Consensus series 10 - Diagnosis of primary open angle glaucoma (Kugler Publications, 2016).
URL
https://patents.google.com/patent/US20190110681A1/en
Description
Optical Texture Analysis of the Inner Retina (US 20190110681)
Learn more about this trial
Glaucoma Screening With Artificial Intelligence
We'll reach out to this number within 24 hrs