Machine Learning Sepsis Alert Notification Using Clinical Data (HindSight P2)
Primary Purpose
Sepsis, Severe Sepsis, Septic Shock
Status
Recruiting
Phase
Phase 2
Locations
United States
Study Type
Interventional
Intervention
HindSight
InSight
Sponsored by
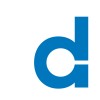
About this trial
This is an interventional other trial for Sepsis focused on measuring Sepsis, Machine learning algorithm, Clinical decision support
Eligibility Criteria
Inclusion Criteria:
- During the study period, all patients over the age of 18 presenting to the emergency department or admitted to an inpatient unit at the participating facilities will automatically be enrolled in the study, until the enrollment target for the study is met
Exclusion Criteria:
- Patients under the age of 18
- Prisoners
Sites / Locations
- Baystate HealthRecruiting
- Cooper University Health CareRecruiting
- Cape Regional Medical CenterRecruiting
Arms of the Study
Arm 1
Arm 2
Arm Type
Experimental
Active Comparator
Arm Label
Experimental
Control
Arm Description
The experimental arm will involve patients monitored by HindSight.
The control arm will involve patients monitored by InSight.
Outcomes
Primary Outcome Measures
Rate of reduction in false alerts
The primary outcome measure of interest will be false alert reduction. Successful completion of Aim 1 will be demonstrated by a positive predictive value (PPV) in a live clinical setting for which the lower bound of the 95% confidence interval meets or exceeds the benchmark from prior retrospective studies. Meeting the retrospective PPV benchmark indicates that prospective CDS quality reflects retrospective CDS quality, and is sufficiently high to reduce alarm fatigue and improve clinical utility. Success of Aim 2 is contingent upon achieving a 15% relative reduction in false alerts when comparing between the two treatment arms (p < 0.05; Fisher's Exact Test).
Secondary Outcome Measures
Full Information
NCT ID
NCT04005001
First Posted
June 28, 2019
Last Updated
April 27, 2022
Sponsor
Dascena
Collaborators
Cape Regional Medical Center, Cooper University Medical Center, Baystate Health, National Institute on Alcohol Abuse and Alcoholism (NIAAA)
1. Study Identification
Unique Protocol Identification Number
NCT04005001
Brief Title
Machine Learning Sepsis Alert Notification Using Clinical Data
Acronym
HindSight P2
Official Title
Using Clinical Treatment Data in a Machine Learning Approach for Sepsis Detection
Study Type
Interventional
2. Study Status
Record Verification Date
April 2022
Overall Recruitment Status
Recruiting
Study Start Date
September 25, 2021 (Actual)
Primary Completion Date
August 31, 2022 (Anticipated)
Study Completion Date
August 31, 2022 (Anticipated)
3. Sponsor/Collaborators
Responsible Party, by Official Title
Sponsor
Name of the Sponsor
Dascena
Collaborators
Cape Regional Medical Center, Cooper University Medical Center, Baystate Health, National Institute on Alcohol Abuse and Alcoholism (NIAAA)
4. Oversight
Studies a U.S. FDA-regulated Drug Product
No
Studies a U.S. FDA-regulated Device Product
No
Data Monitoring Committee
No
5. Study Description
Brief Summary
Machine learning is a powerful method to create clinical decision support (CDS) tools, when training labels reflect the desired alert behavior. In our Phase I work for this project, we developed HindSight, an encoding software that was designed to examine discharged patients' electronic health records (EHRs), identify clinicians' sepsis treatment decisions and patient outcomes, and pass those labeled outcomes and treatment decisions to an online algorithm for retraining of our machine-learning-based CDS tool for real-time sepsis alert notification, InSight. HindSight improved the performance of InSight sepsis alerts in retrospective work. In this study, we propose to assess the clinical utility of HindSight by conducting a multicenter prospective randomized controlled trial (RCT) for more accurate sepsis alerts.
Detailed Description
We will evaluate the performance of HindSight in a randomized controlled trial (RCT). HindSight is a novel encoding software designed to optimize alerts for sepsis alert notification. HindSight identifies clinicians' sepsis-related decisions in the electronic health records of former patients and passes those events to InSight, thus supplying InSight with labeled examples of true positive sepsis cases for retraining. In our retrospective work, we have shown that HindSight enables InSight to adapt to site-specific deviations of real-world clinical deployment by successfully reducing false and irrelevant alarms, without human supervision. The goal of this project is to demonstrate that the retrospective success of HindSight can be successfully translated to live clinical environments. In our Phase I work, HindSight achieved an area under the receiver-operating characteristic (AUROC) of 0.899, 0.831 and 0.877 for clinician sepsis evaluation, treatment, and onset, respectively. By using an online learning algorithm to incorporate HindSight-labeled data into the InSight predictor, we showed that the online-trained InSight can adapt to the HindSight-labeled data and outperform both baseline and periodically re-trained versions of InSight (p < 0.05). In Aim 1, we will prospectively validate HindSight's performance on real-time patient data streams in three diverse hospitals non-interventionally. In Aim 2, we will evaluate the effect of the tool in a prospective, interventional RCT. HindSight will first be evaluated by live deployment at four academic and community hospitals, during which time it will not provide alerts of future sepsis onset. Following any necessary algorithm optimization arising from live hospital validation, we will perform an RCT to evaluate reductions in false alerts from InSight trained on HindSight sepsis labels (experimental arm), compared to InSight trained on gold standard Sepsis-3 labels (control arm).
6. Conditions and Keywords
Primary Disease or Condition Being Studied in the Trial, or the Focus of the Study
Sepsis, Severe Sepsis, Septic Shock
Keywords
Sepsis, Machine learning algorithm, Clinical decision support
7. Study Design
Primary Purpose
Other
Study Phase
Phase 2
Interventional Study Model
Parallel Assignment
Masking
ParticipantCare ProviderInvestigator
Allocation
Randomized
Enrollment
37986 (Anticipated)
8. Arms, Groups, and Interventions
Arm Title
Experimental
Arm Type
Experimental
Arm Description
The experimental arm will involve patients monitored by HindSight.
Arm Title
Control
Arm Type
Active Comparator
Arm Description
The control arm will involve patients monitored by InSight.
Intervention Type
Other
Intervention Name(s)
HindSight
Other Intervention Name(s)
HindSight-Clinical decision support (CDS) system for sepsis alert notification
Intervention Description
HindSight will examine the dynamic trends of clinical measurements taken from a patient's EHR and analyzes correlations between vital signs to alert for the onset of sepsis.This machine learning based tool is optimized by encoder and utilizes periodic retraining to improve its performance over time.
Intervention Type
Other
Intervention Name(s)
InSight
Intervention Description
Compared to the ability of the InSight software's recognition of sepsis onset to HindSight's performance. The study determines if the HindSight software has equivalent or better performance than the InSight software.
Primary Outcome Measure Information:
Title
Rate of reduction in false alerts
Description
The primary outcome measure of interest will be false alert reduction. Successful completion of Aim 1 will be demonstrated by a positive predictive value (PPV) in a live clinical setting for which the lower bound of the 95% confidence interval meets or exceeds the benchmark from prior retrospective studies. Meeting the retrospective PPV benchmark indicates that prospective CDS quality reflects retrospective CDS quality, and is sufficiently high to reduce alarm fatigue and improve clinical utility. Success of Aim 2 is contingent upon achieving a 15% relative reduction in false alerts when comparing between the two treatment arms (p < 0.05; Fisher's Exact Test).
Time Frame
Through study completion, human subjects involvement will occur for an average of eight months
10. Eligibility
Sex
All
Minimum Age & Unit of Time
18 Years
Accepts Healthy Volunteers
Accepts Healthy Volunteers
Eligibility Criteria
Inclusion Criteria:
During the study period, all patients over the age of 18 presenting to the emergency department or admitted to an inpatient unit at the participating facilities will automatically be enrolled in the study, until the enrollment target for the study is met
Exclusion Criteria:
Patients under the age of 18
Prisoners
Central Contact Person:
First Name & Middle Initial & Last Name or Official Title & Degree
Jana Hoffman, PhD
Phone
2158806619
Email
jana@dascena.com
First Name & Middle Initial & Last Name or Official Title & Degree
Gina Barnes, MPH
Phone
2158806619
Email
gbarnes@dascena.com
Overall Study Officials:
First Name & Middle Initial & Last Name & Degree
Jana Hoffman, PhD
Organizational Affiliation
Dascena
Official's Role
Principal Investigator
Facility Information:
Facility Name
Baystate Health
City
Springfield
State/Province
Massachusetts
ZIP/Postal Code
01199
Country
United States
Individual Site Status
Recruiting
Facility Contact:
First Name & Middle Initial & Last Name & Degree
Gregory Braden
Facility Name
Cooper University Health Care
City
Camden
State/Province
New Jersey
ZIP/Postal Code
08103
Country
United States
Individual Site Status
Recruiting
Facility Contact:
First Name & Middle Initial & Last Name & Degree
Sharad Patel
First Name & Middle Initial & Last Name & Degree
Snehal Gandhi
Facility Name
Cape Regional Medical Center
City
Cape May
State/Province
New Jersey
ZIP/Postal Code
08210
Country
United States
Individual Site Status
Recruiting
Facility Contact:
First Name & Middle Initial & Last Name & Degree
Andrea McCoy
12. IPD Sharing Statement
Plan to Share IPD
No
Citations:
PubMed Identifier
28638239
Citation
Desautels T, Calvert J, Hoffman J, Mao Q, Jay M, Fletcher G, Barton C, Chettipally U, Kerem Y, Das R. Using Transfer Learning for Improved Mortality Prediction in a Data-Scarce Hospital Setting. Biomed Inform Insights. 2017 Jun 12;9:1178222617712994. doi: 10.1177/1178222617712994. eCollection 2017.
Results Reference
background
PubMed Identifier
27253619
Citation
Calvert J, Mao Q, Rogers AJ, Barton C, Jay M, Desautels T, Mohamadlou H, Jan J, Das R. A computational approach to mortality prediction of alcohol use disorder inpatients. Comput Biol Med. 2016 Aug 1;75:74-9. doi: 10.1016/j.compbiomed.2016.05.015. Epub 2016 May 24.
Results Reference
background
PubMed Identifier
27026611
Citation
Calvert JS, Price DA, Barton CW, Chettipally UK, Das R. Discharge recommendation based on a novel technique of homeostatic analysis. J Am Med Inform Assoc. 2017 Jan;24(1):24-29. doi: 10.1093/jamia/ocw014. Epub 2016 Mar 28.
Results Reference
background
PubMed Identifier
27699003
Citation
Calvert J, Mao Q, Hoffman JL, Jay M, Desautels T, Mohamadlou H, Chettipally U, Das R. Using electronic health record collected clinical variables to predict medical intensive care unit mortality. Ann Med Surg (Lond). 2016 Sep 6;11:52-57. doi: 10.1016/j.amsu.2016.09.002. eCollection 2016 Nov.
Results Reference
background
PubMed Identifier
29435343
Citation
Shimabukuro DW, Barton CW, Feldman MD, Mataraso SJ, Das R. Effect of a machine learning-based severe sepsis prediction algorithm on patient survival and hospital length of stay: a randomised clinical trial. BMJ Open Respir Res. 2017 Nov 9;4(1):e000234. doi: 10.1136/bmjresp-2017-000234. eCollection 2017.
Results Reference
background
Learn more about this trial
Machine Learning Sepsis Alert Notification Using Clinical Data
We'll reach out to this number within 24 hrs