Machine Learning for Risk Stratification in the Emergency Department (MARS-ED) (MARS-ED)
Primary Purpose
Acute Pain, Emergencies
Status
Recruiting
Phase
Not Applicable
Locations
Netherlands
Study Type
Interventional
Intervention
RISK-INDEX
Sponsored by
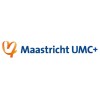
About this trial
This is an interventional diagnostic trial for Acute Pain focused on measuring machine learning, risk stratification, pilot
Eligibility Criteria
Inclusion Criteria:
- Adult, defined as ≥ 18 years of age
- Assessed and treated by an internal medicine specialist (gastroenterologists included) in the ED
- Willing to give written consent, either directly or after deferred consent procedure (see section 11.2).
Exclusion Criteria:
- <4 different laboratory results available (hematology or clinical chemistry) within the first two hours of the ED visit (calculation ML prediction score otherwise not possible)
- Unwilling to provide written consent, either directly or after deferred consent procedure (see section 11.2).
Sites / Locations
- Maastricht University Medical Centre+Recruiting
Arms of the Study
Arm 1
Arm 2
Arm Type
No Intervention
Experimental
Arm Label
Standard care
RISKINDEX
Arm Description
Routine clinical care. Physicians will actively be asked to self-report their clinical impression of each included patient and policy will be monitored.
Routine clinical care. Physicians will actively be asked to self-report their clinical impression of each included patient and policy will be monitored. In the intervention group, physicians will be presented with the RISKINDEX. Subsequently, self-report will again be initiated to evaluate the physicians' response to the ML score and possible policy changes due to the intervention.
Outcomes
Primary Outcome Measures
RISK-INDEX performance
Discriminatory performance of ML risk score to predict 31-day mortality. This will be calculated using an area under the receiver operating characteristic curves (AUC).
Policy changes
Policy changes after presentation of RISK-INDEX. This will be assessed by a filled out questionnaire by the physician where they state whether a policy change has been made as a result of the RISK-INDEX outcome.
Secondary Outcome Measures
Full Information
NCT ID
NCT05497830
First Posted
July 19, 2022
Last Updated
November 2, 2022
Sponsor
Maastricht University Medical Center
1. Study Identification
Unique Protocol Identification Number
NCT05497830
Brief Title
Machine Learning for Risk Stratification in the Emergency Department (MARS-ED)
Acronym
MARS-ED
Official Title
Machine Learning for Risk Stratification in the Emergency Department: A Pilot Clinical Trial
Study Type
Interventional
2. Study Status
Record Verification Date
August 2022
Overall Recruitment Status
Recruiting
Study Start Date
September 12, 2022 (Actual)
Primary Completion Date
January 1, 2024 (Anticipated)
Study Completion Date
March 1, 2024 (Anticipated)
3. Sponsor/Collaborators
Responsible Party, by Official Title
Sponsor
Name of the Sponsor
Maastricht University Medical Center
4. Oversight
Studies a U.S. FDA-regulated Drug Product
No
Studies a U.S. FDA-regulated Device Product
No
Data Monitoring Committee
Yes
5. Study Description
Brief Summary
Rationale
Identifying emergency department (ED) patients at high and low risk shortly after admission could help decision-making regarding patient care. Several clinical risk scores and triage systems for stratification of patients have been developed, but often underperform in clinical practice. Moreover, most of these risk scores only have been diagnostically validated in an observational cohort, but never have been evaluated for their actual clinical impact. In a recent retrospective study that was conducted in the Maastricht University Medical Center (MUMC+), a novel clinical risk score, the RISKINDEX, was introduced that predicted 31-day mortality of sepsis patients presenting to an ED. The RISKINDEX hereby also outperformed internal medicine specialists. Observational follow-up studies underlined the potential of the risk score. However, it remains unknown to what extent these models have any beneficial value when it is actually implemented in clinical practice.
Objective
To determine the diagnostic accuracy, policy changes and clinical impact of the RISKINDEX as basis to conduct a large scale, multi-center randomised trial.
Study design
The MARS-ED study is designed as a multi-center, randomized, open-label, non-inferiority pilot clinical trial.
Study population
Adult patients who are assessed and treated by an internal medicine specialist in the ED of whom a minimum of 4 different laboratory results (hematology or clinical chemistry, required for calculation of ML risk score) are available within the first two hours of the ED visit.
Intervention
Physicians will be presented with the ML risk score (the RISKINDEX) of the patients they are actively treating, directly after assessment of regular diagnostics has taken place.
Main study parameters
Primary
- Diagnostic accuracy, policy changes and clinical impact of a novel clinical risk score (the RISKINDEX)
Secondary
Policy changes due to presentation of ML score (treatment policy, requesting ancillary investigations, treatment restrictions (i.e., no intubation or resuscitation)
Intensive care (ICU) and medium care (MC) admission
Length of admission
Mortality within 31 days
Readmission
Patient preference
Feasibility of novel clinical risk score
6. Conditions and Keywords
Primary Disease or Condition Being Studied in the Trial, or the Focus of the Study
Acute Pain, Emergencies
Keywords
machine learning, risk stratification, pilot
7. Study Design
Primary Purpose
Diagnostic
Study Phase
Not Applicable
Interventional Study Model
Parallel Assignment
Masking
None (Open Label)
Allocation
Randomized
Enrollment
2070 (Anticipated)
8. Arms, Groups, and Interventions
Arm Title
Standard care
Arm Type
No Intervention
Arm Description
Routine clinical care. Physicians will actively be asked to self-report their clinical impression of each included patient and policy will be monitored.
Arm Title
RISKINDEX
Arm Type
Experimental
Arm Description
Routine clinical care. Physicians will actively be asked to self-report their clinical impression of each included patient and policy will be monitored. In the intervention group, physicians will be presented with the RISKINDEX. Subsequently, self-report will again be initiated to evaluate the physicians' response to the ML score and possible policy changes due to the intervention.
Intervention Type
Other
Intervention Name(s)
RISK-INDEX
Intervention Description
Presentation of RISKINDEX to the physician after approximately 2 hours. The ML RISKINDEX is a prediction model based on laboratory data from the ED. It is based on date of birth, sex and at least four laboratory data which are sampled within the first two hours of the ED visit. Laboratory data that are used as input include samples that are commonly drawn in patients that require treatment from an internal medicine physician, such as urea, albumin, C-reactive protein (CRP), lactate and bilirubin.
Primary Outcome Measure Information:
Title
RISK-INDEX performance
Description
Discriminatory performance of ML risk score to predict 31-day mortality. This will be calculated using an area under the receiver operating characteristic curves (AUC).
Time Frame
31 days
Title
Policy changes
Description
Policy changes after presentation of RISK-INDEX. This will be assessed by a filled out questionnaire by the physician where they state whether a policy change has been made as a result of the RISK-INDEX outcome.
Time Frame
As soon as RISK-INDEX score is presented
10. Eligibility
Sex
All
Minimum Age & Unit of Time
18 Years
Accepts Healthy Volunteers
Accepts Healthy Volunteers
Eligibility Criteria
Inclusion Criteria:
Adult, defined as ≥ 18 years of age
Assessed and treated by an internal medicine specialist (gastroenterologists included) in the ED
Willing to give written consent, either directly or after deferred consent procedure (see section 11.2).
Exclusion Criteria:
<4 different laboratory results available (hematology or clinical chemistry) within the first two hours of the ED visit (calculation ML prediction score otherwise not possible)
Unwilling to provide written consent, either directly or after deferred consent procedure (see section 11.2).
Central Contact Person:
First Name & Middle Initial & Last Name or Official Title & Degree
Steven Meex, PhD
Phone
+31 (0)43 3874709
Email
steven.meex@mumc.nl
First Name & Middle Initial & Last Name or Official Title & Degree
Patricia Stassen, PhD, MD
Phone
+31 (0)43 3875005
Email
p.stassen@mumc.nl
Facility Information:
Facility Name
Maastricht University Medical Centre+
City
Maastricht
State/Province
Limburg
ZIP/Postal Code
6229 HX
Country
Netherlands
Individual Site Status
Recruiting
Facility Contact:
First Name & Middle Initial & Last Name & Degree
Steven Meex, PhD
Phone
+31 (0)43 3874709
Email
steven.meex@mumc.nl
First Name & Middle Initial & Last Name & Degree
Patricia Stassen, PhD, MD
Phone
+31 (0)43 3875005
Email
p.stassen@mumc.nl
12. IPD Sharing Statement
Plan to Share IPD
No
Citations:
PubMed Identifier
33465096
Citation
van Doorn WPTM, Stassen PM, Borggreve HF, Schalkwijk MJ, Stoffers J, Bekers O, Meex SJR. A comparison of machine learning models versus clinical evaluation for mortality prediction in patients with sepsis. PLoS One. 2021 Jan 19;16(1):e0245157. doi: 10.1371/journal.pone.0245157. eCollection 2021.
Results Reference
result
Links:
URL
https://www.medrxiv.org/content/10.1101/2020.11.25.20238386v2
Description
Explainable Machine Learning models for Rapid Risk Stratification in the Emergency Department: A multi-center study
Learn more about this trial
Machine Learning for Risk Stratification in the Emergency Department (MARS-ED)
We'll reach out to this number within 24 hrs